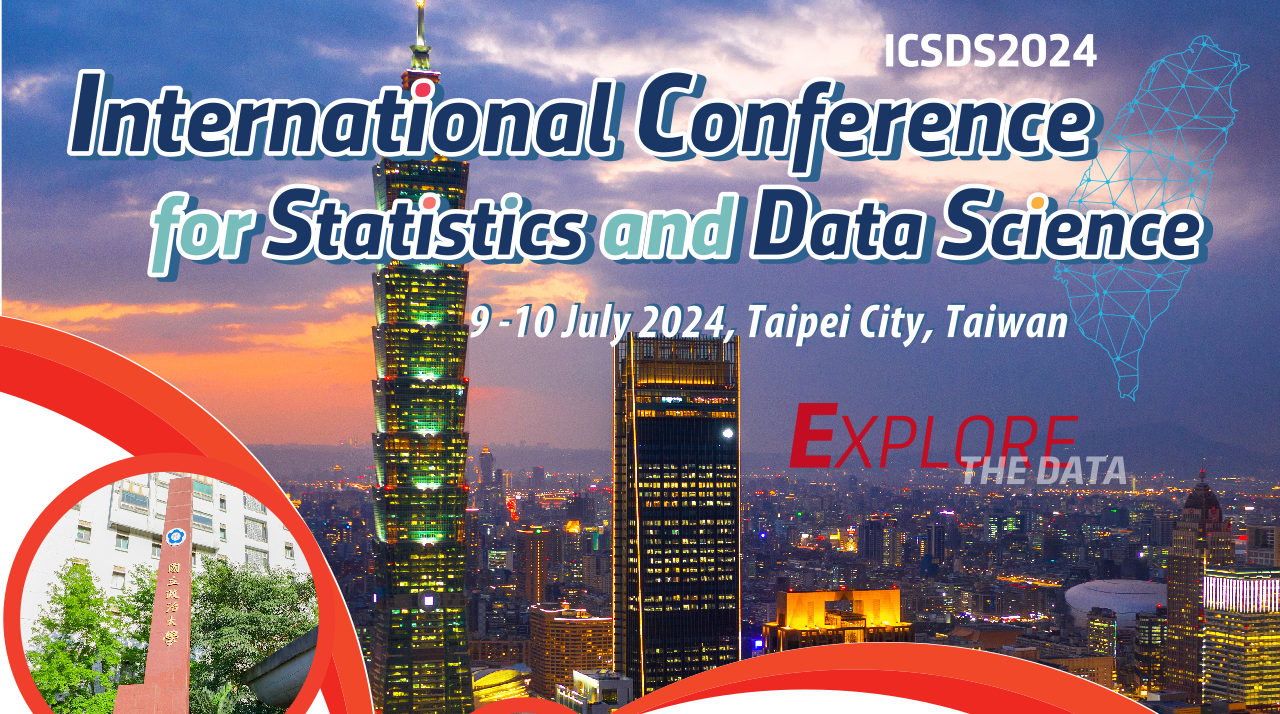
The first International Conference for Statistics and Data Science (ICSDS 2023) was a collaborative effort between National Yang Ming Chiao Tung University, the Taiwan Chapter of the International Chinese Statistical Association, the Institute of Statistical Science at Academia Sinica, and the Chinese Institute of Probability and Statistics. Building on its success, the second ICSDS is set for July 9-10, 2024, hosted by Department of Statistics at National Chengchi University, the Institute of Statistics at NYCU, and the Taiwan Chapter of ICSA. The conference will mirror the scale of its predecessor, attracting international experts from the United States, China, Taiwan, Canada, the UK, and Italy. It promises an agenda rich in keynote speeches and symposiums, covering diverse aspects of statistics and data science. The two-day event will explore topics like Complex Data in Finance, Text Mining, Bioinformatics, Big Data, Industrial Statistics, Medical and Spatial Statistics, Clinical Trial Design, Time Series, Biostatistics, Actuarial Statistics, Machine Learning, Dimension Reduction, Sparse Modeling, Stochastic Processes, Bayesian Inference, and Nonparametric Statistics. Aimed at promoting the field of statistics, the ICSDS seeks to enhance international academic collaboration, create cooperative opportunities, and drive the progress and development of statistical sciences. This academic forum is expected to be a nexus for sharing innovative ideas, methodologies, and fostering advancements in statistics and data science.
Keynote Speakers
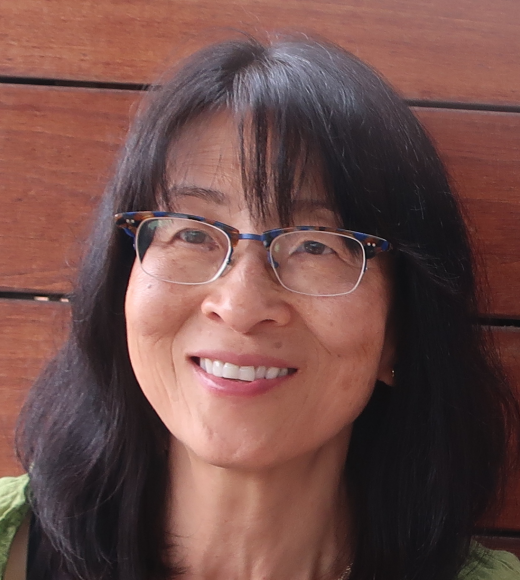
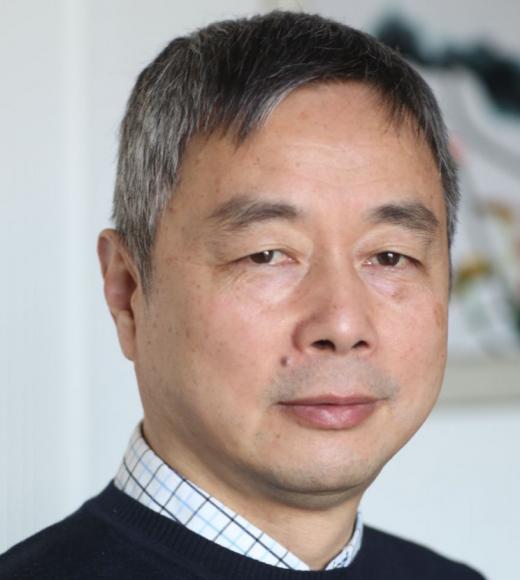
Jane-Ling Wang (王建玲)
Distinguished Professor, Department of Statistics, University of California, Davis, U.S.A. (中研院院士, Fellow of IMS, Fellow of ASA, Elected member of ISI)
Title:Deep Learning for Censored Survival Data
Qiwei Yao (姚琦偉)
Professor, Department of Statistics, London School of Economics and Political Science, U.K. (Fellow of IMS, Fellow of ASA, Elected member of ISI)
Title:Autoregressive Networks with Stylized Features
News
- 2024/07/31: Photos for 2024/07/10
- 2024/07/30: Photos for 2024/07/09.
- 2024/07/07: ICSDS2024 Programme Book (pdf).
- 2024/07/06: Pre-Conference Notice (行前通知). (pdf)
- 2024/07/06: Transportation Information (搭乘大眾交通工具或自行開車前來). (pdf)
- 2024/07/06: Conference Programme (研討會議程(全英文)). (pdf)
- 2024/07/06: Attendees (參加者名冊及報到序號(依身份別)). (pdf)
- 2024/07/03: The tentative conference program has been updated. (pdf)
- 2024/06/20: The tentative conference program has been updated. (pdf)
- 2024/05/16: The tentative conference program has been updated. (pdf)
- 2024/04/28: The tentative conference program has been announced. (pdf)
- 2024/04/06: The list of speakers is updated.
- 2024/03/01: Online registration is now open.
- 2024/01/23: Online registration is scheduled to open on 2024/03/01.
- 2023/12/25: The Conference Poster. (Updated)
- 2023/12/25:The ICSDS2024 website is announced.